How neural networks can help us gain a deeper understanding of financial markets
A new research project at Aarhus University will use Bayesian Neural Networks to model, analyse and understand trading behaviour in the world of finance. The researchers behind the project expect the technology to revolutionise our understanding of financial data.
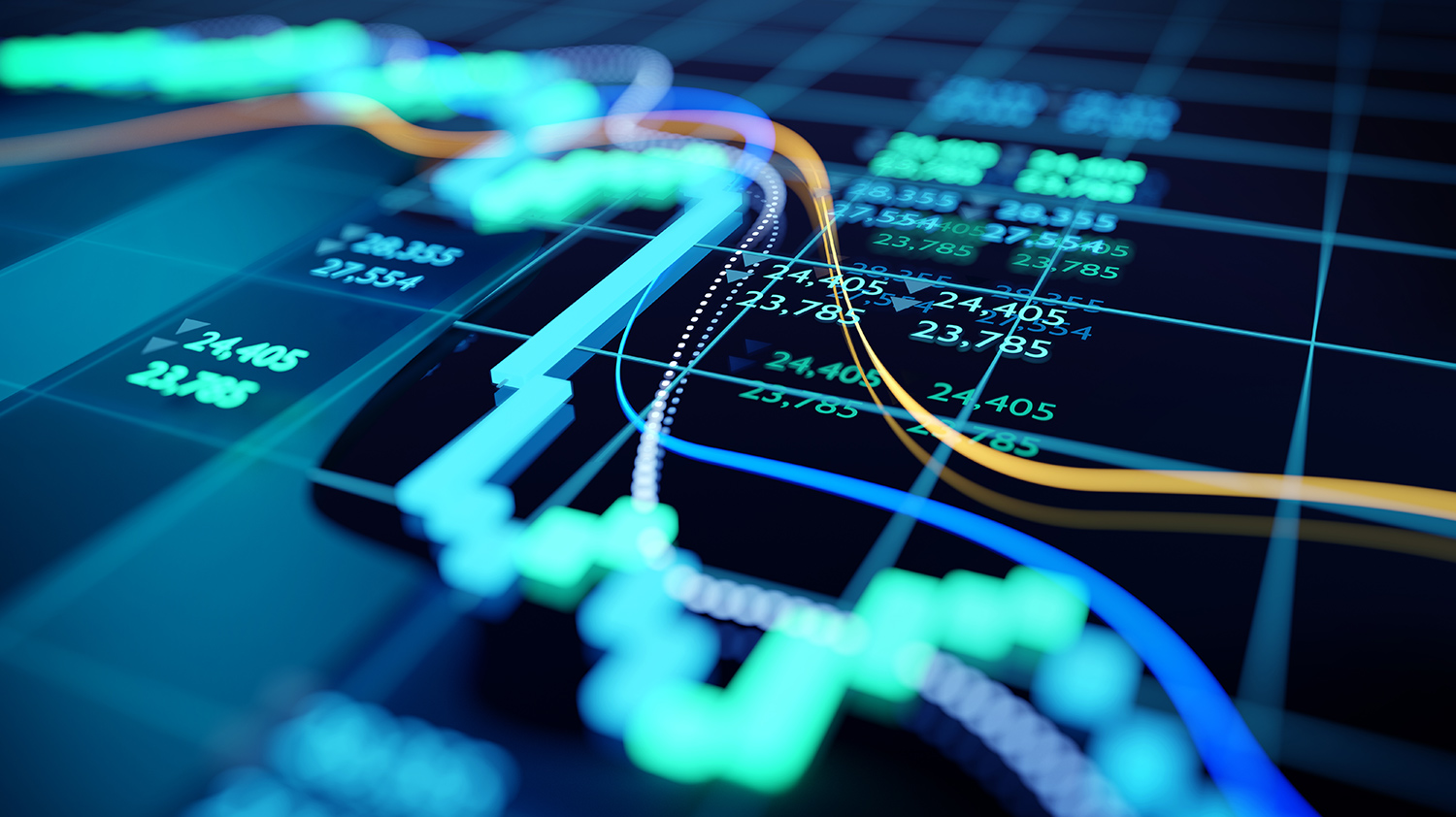
For the first time ever, a team of artificial intelligence researchers and experts will use Bayesian Neural Networks (BNN), a kind of deep machine learning algorithm, to analyse, model and understand causal relationships within trading on global financial markets.
BNN is a quantum leap in the application of digital tools for modern, multi-dimensional analysis of complex and unstructured financial data, where ordinary econometric methods often fail:
"People who work with econometrics and economics take a keen interest in probability distribution. But the machine learning techniques used so far are inadequate, because they’re unable to cope with the complexity of the financial world. BNN can revolutionise our understanding of financial data, because the algorithm can tell us about the probability of a given outcome," says Martin Magris, a Marie Sk?odowska-Curie Individual Fellow at the Department of Engineering and a member of the Machine Learning and Computational Intelligence research group headed by Associate Professor Alexandros Iosifidis.
Bayesian Neural Networks are a kind of machine learning model equipped with what we can describe as common sense. BNN combines traditional training data from machine learning with integrated statistical knowledge and experience of the relevant domain obtained by data.
This enables the machine to provide information related to probability distributions based on quite extensive and complex data and thereby significantly improve the econometric modelling processes, analyses and insight into the complexity of the financial markets.
"Today, financial markets are based entirely on computers and algorithms. The need for machine learning techniques to understand data is greater than ever before. This project will provide measurable improvements compared with state-of-the-art technologies used to process high-frequency financial data within volatility modelling, prediction of stock price movements and analysis of mutual dependency between stock prices," says Martin Magris.
The research project is called BNNmetrics and is headed by Martin Magris. Funding for the project, amounting to DKK 2.8 million, comes from the Marie Sk?odowska-Curie Actions within Horizon 2020, the EU Framework Programme for Research and Innovation.
Contact
Postdoc Martin Magris
Mail: magris@eng.au.dk
Tel.: 71396616