Fast & furious: Autonomous drone racing raises the bar for artificial intelligence
Today, drones can autonomously carry out various tasks with zero or minimum involvement by human operators. However, they need to fly as slow as possible to sense their environment and plan future actions. But what if drones need to fly fast like in a manual drone race where speed, agility, and performance are paramount? A team of researchers from Aarhus University have just taken a step forward.
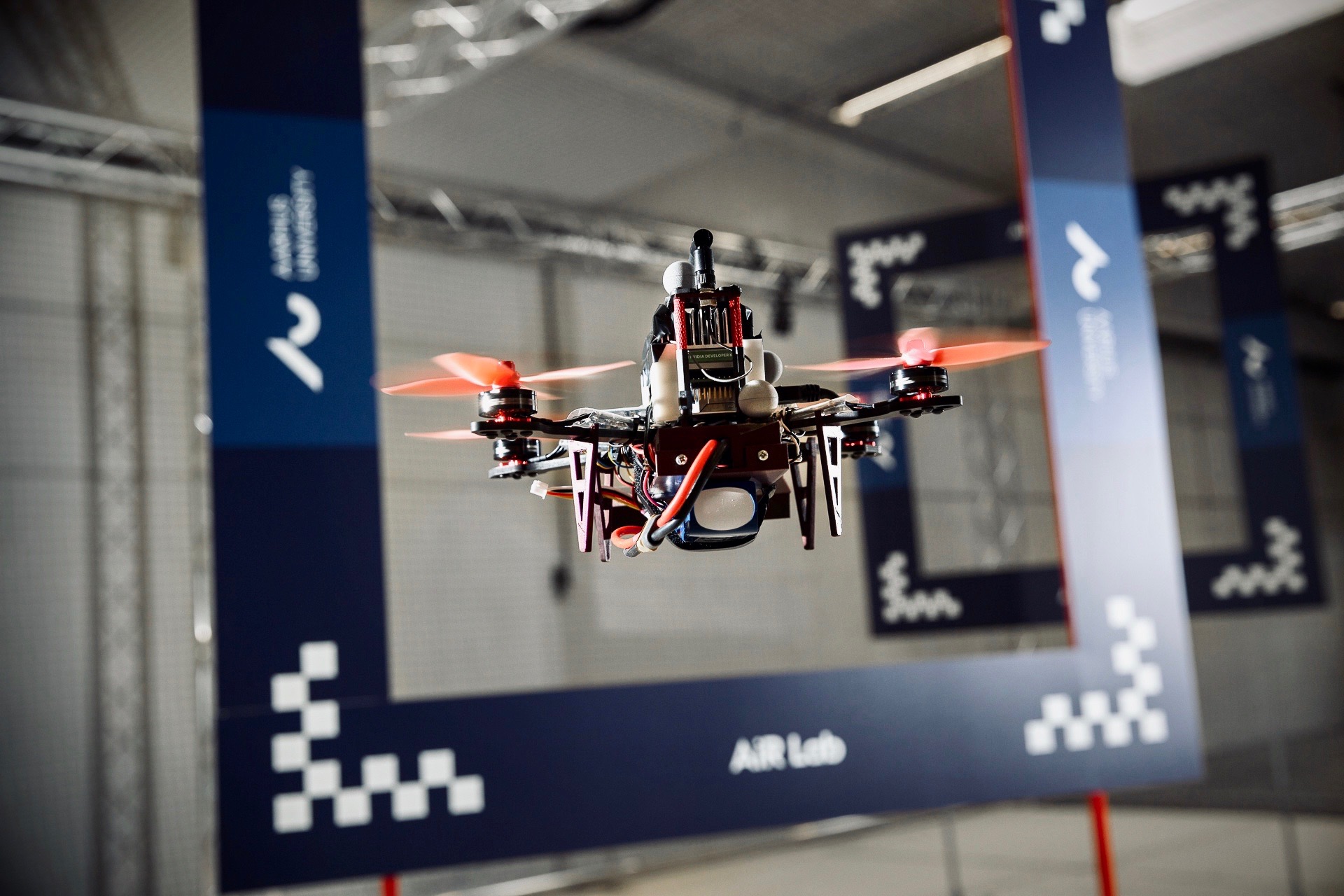
One of the major Gordian knots in the field of autonomous transportation is for artificial intelligence (AI) to perceive and understand the world around it, to react and manoeuvre in time. This holds true, no matter whether the AI controls a car, a ship, a plane, or even an aerial taxi or a drone.
One of the great hopes in untangling this knot is drone racing. Drone racing is seeing rapid growth all over the world, and it entails using a VR headset to steer a drone through gates in an obstacle course as quickly as possible.However, AI researchers are interested in autonomous drone racing, where only the AI guides the drone through the gates on the course.
If you want to win a drone race, it is important to fly fast and safe while avoiding obstacles. And that means the drone has to be light, small and agile.
This is where computer vision comes in. If the drone has to be light, small and agile, there is not space for many sensors to monitor the landscape as the drone passes, and there is nowhere near enough energy in the drone's small batteries to keep up with computationally heavy neural networks requiring large amounts of energy to make the AI react to inputs.
So, if we can develop efficient AI algorithms that can manoeuvre a drone through gates on an obstacle course in the same way as humans can, this will have far-reaching consequences for a great many applications. For example, within robot technology.
"We're looking for an AI to react to unknown terrain, while at the same time using the least possible power and the fewest possible sensors. And autonomous drone racing is the best way to push the boundaries of what's possible," says Associate Professor Erdal Kayacan from Aarhus University.
The dream is to design a fully autonomous drone which can beat the best human pilot in a race, using sophisticated AI techniques. This long-term goal is challenging, as researchers encounter problems to the existing problems in robotics, e.g. motion blur coming from cameras, nonlinear behaviour of the drone at high speeds and limited computation power on the drone.
(The article continues below the picture)
Associate Professor Erdal Kayacan, Aarhus University, is an expert in artificial intelligence and drones. Photo: Lars Kruse, AU Foto.
Erdal Kayacan is in charge of the Artificial Intelligence in Robotics (AiR) research group at the university, and they are pushing the boundaries of what AI can do.
The team has just developed a new data generation methodology that will form the basis for faster and more efficient training of AI.
"Generating data sets with information about the actual surroundings, so-called ground truth, is just as important today as suggesting completely new methods for machine learning," says the associate professor.
He explains that, currently, there are basically three ways of generating these data sets. You can collect data from the real world, collect it from simulations, or use a combination of the two.
The first method (collecting the data from the real world) generates real images of real gates in real environments. But this method requires manual labelling of the gates on each training image, which is time-consuming. When an automatic labelling method is preferred, this method requires a very accurate position of the gate with respect to the drone which is not always available.
On the other hand, in the second method (collecting data from simulation), everything is precisely defined in the simulated environment. But the main drawback of this method is the difficulty to create photorealistic images of the environment.
And then there is the third method that combines the advantages of the previous two. This method has been developed by the research team themselves, and the team has just published an article about it at the WCCI 2020, World Congress of Computational Intelligence, which took place at the end of July.
(The article continues below the video)
Presentation of the novel method at the World Congress of Computational Intelligence 2020.
"We call the method 'semi-synthetic', as we combine real background images with randomised 3D renderings of the gates that the drone has to pass through. This means that we can very quickly calculate ground truth for practically every measurement, and this makes it quick to train the AI in an unlimited amount of exercises," says Erdal Kayacan.
The AiR research group at Aarhus University is far from the only research group working with drones. And even though it may sound like a game, the associate professor hopes that the techniques for computer vision being developed in connection with autonomous drone racing will find wide use in AI.
In addition to Associate Professor Erdal Kayacan, visiting PhD student Andriy Sarabakha from Nanyang Technological University and MSc degree student Théo Morales have contributed to the novel methodology. The article, "Image Generation for Efficient Neural Network Training in Autonomous Drone Racing", was published at WCCI 2020.